The field of artificial intelligence has seen tremendous growth and advancement in recent years, with researchers and scientists working hard to develop new technologies and applications.
One field that has seen considerable progress is Generative AI, which refers to the use of machine learning algorithms to create new data.
In this article, we will explore the concept of Generative AI and its impact on businesses.
What is Generative AI?
Generative AI is the process of using machine-learning algorithms to produce new content. For instance, music, photographs, and even written content can all be created through generative AI.
The key to this technology is the ability to create completely new content that is distinct from any pre-existing data. In other words, the AI is not merely duplicating existing content but creating something entirely new from scratch.
How does Generative AI work?
Generative AI leverages machine learning techniques to learn from data and generate new content that exhibits similar characteristics to the input data. Its operation involves training on large datasets, learning representations of the data, generating new content, optimizing model parameters, and customization for specific applications.
Here’s an overview of how it operates:
Data Input and Training
Generative AI models are trained on large datasets containing examples of the type of content they are intended to generate. For example, a text generation model like GPT-3 is trained on vast amounts of text data from the internet.
During training, the model learns patterns, structures, and relationships within the input data.
Learning and Representation
The model learns a representation of the input data, capturing features and statistical regularities to understand the underlying patterns. This learning process involves adjusting the model’s internal parameters to minimize the difference between the generated content and the original data.
Generation Process
Once trained, the Generative AI model can produce new content by sampling from the learned representation. For instance, a language generation model can predict and generate coherent sentences or paragraphs based on a given prompt or context.
Feedback and Optimization
Generative AI models are often refined and optimized through iterative feedback loops, adjusting the model’s parameters to improve the quality of the generated outputs. This process involves validation against human judgment and continuous learning from new data.
Applications of Generative AI in Business
Generative AI has several applications in the business world. For instance, it could be useful to the marketing and advertising teams, which can use it to create compelling advertisements automatically.
For instance, GPT-3 can generate copy that’s engaging and convincing. Entertainment companies could use it to create custom music playlists and video recommendations for each user based on their preferences.
In fashion retail, generative algorithms can be applied to the clothing design process. Computer Vision is capable of generating designs from images and data. This is particularly helpful in the initial stages of designing when creative ideas are more important than practicality.
Additionally, machine learning algorithms can predict future trends in the market, such as colours or designs that will become popular, creating an edge in our competitive industry.
Examples of Generative AI
Here are some examples of Generative AI models that have made a significant impact:
1. GPT-3 (Generative Pre-trained Transformer 3)
Developed by OpenAI, GPT-3 is one of the most advanced language generation models. It can generate human-like text based on the input prompt, perform language translation, answer questions, and even create poetry.
Its versatility and ability to generate coherent and contextually relevant text have made it a game-changer in natural language processing and content creation.
2. DALL-E
Also created by OpenAI, DALL·E is a neural network that can generate images from textual descriptions. It can conceptualize and create images based on the input provided, demonstrating a remarkable capability for creative visual synthesis.
3. StyleGAN
This model, developed by NVIDIA, specializes in generating high-quality images, particularly human faces. It is capable of creating realistic and diverse facial images, showcasing the potential of Generative AI in visual content creation and art generation.
4. Magenta
Developed by Google, Magenta focuses on generating music and art using machine learning. It has produced compositions and artworks that are indistinguishable from those created by humans, demonstrating the exciting potential of Generative AI in the domain of artistic expression.
These examples illustrate the wide-ranging capabilities of Generative AI across different modalities, including language, visual content, music, and art, showcasing its transformative potential in various creative and practical applications.
Challenges of Generative AI
While Generative AI is a promising technology with significant potential for industry use, there are some challenges. For instance, there is the problem of making sure the AI produces desirable data.
The Generative AI can produce useless data or even worse produce data that is harmful.
Furthermore, this technology can be expensive for businesses that require processing a vast amount of data.
How do I apply generative AI?
You can apply Generative AI in various ways across different industries and domains. Here are some practical applications:
1. Content Creation
Use Generative AI to automate and optimize content creation processes, including generating articles, product descriptions, social media posts, and advertising copy. Companies can leverage models like GPT-3 for natural language generation to streamline content production.
2. Creative Design
Employ Generative AI for creative design tasks such as generating artwork, designing visual elements, creating custom graphics, and producing unique patterns. Models like DALL·E can be utilized for image synthesis based on textual descriptions.
3. Personalized Recommendations
Implement Generative AI to deliver personalized recommendations to users, such as customized product suggestions, tailored music playlists, and individualized content based on user preferences and behavior.
4. Prototyping and Simulation
Use Generative AI for rapid prototyping, design optimization, and simulation in fields like architecture, engineering, and manufacturing. It can assist in generating and evaluating design variations and optimizing parameters for complex systems.
5. Healthcare and Life Sciences
Apply Generative AI for drug discovery, protein design, medical image analysis, and patient-specific treatment generation. Models can aid in creating novel pharmaceutical compounds and optimizing treatment plans.
6. Fashion and Retail
Utilize Generative AI for clothing and fashion design, automated trend analysis, and virtual try-on experiences. Computer vision and generative algorithms can facilitate the creation of unique designs and personalized shopping experiences.
7. Entertainment and Gaming
Integrate Generative AI in the creation of interactive narratives, character design, procedural content generation, and immersive gaming experiences. This can enhance user engagement and enable dynamic content generation.
8. Humanitarian and Environmental Impact
Leverage Generative AI for addressing humanitarian and environmental challenges, such as optimizing resource allocation, predicting natural disasters, and generating insights for sustainable development.
When applying Generative AI, it’s crucial to consider ethical implications, data privacy, and potential biases in the generated content. Additionally, organizations should invest in robust training, validation, and monitoring processes to ensure the quality and integrity of the AI-generated outputs.
Limitations of Generative AI
Generative AI, while powerful and innovative, has several limitations that should be taken into consideration:
1. Data Dependency
Generative AI heavily relies on the quality and diversity of the training data. Biases, inaccuracies, or limitations present in the training data can manifest in the generated content, potentially perpetuating or amplifying existing biases and shortcomings.
2. Ethical Concerns
The ability of Generative AI to generate highly convincing content raises ethical concerns, particularly regarding the generation of misinformation, fake media, and deceptive content. It requires thoughtful consideration of responsible usage and potential misuse.
3. Computational Resources
Training and deploying sophisticated Generative AI models often demand substantial computational resources, including high-performance hardware and significant energy consumption. This can pose challenges for smaller organizations or individuals with limited resources.
4. Interpretability and Explainability
Understanding and interpreting the decision-making process of Generative AI models can be challenging due to their complexity and lack of transparency. This limits the ability to explain the rationale behind generated outputs.
5. Overfitting and Quality Control
Generative AI models can be prone to overfitting, where they produce content that closely matches the training data but lacks generalization to new inputs. Quality control mechanisms are essential to ensure the reliability and relevance of generated content.
6. Legal and Regulatory Considerations
The emergence of Generative AI raises legal and regulatory questions, such as intellectual property rights, copyright infringement, and the authenticity of AI-generated content. Clear guidelines and policies are needed to address these matters.
7. Contextual Understanding and Common Sense
Generative AI models may struggle with contextual understanding and common sense reasoning, leading to outputs that lack coherence or fail to grasp nuanced concepts, particularly in complex or ambiguous scenarios.
While these limitations highlight the challenges associated with Generative AI, ongoing research and development efforts are focused on addressing these issues to enhance the reliability, responsibility, and applicability of Generative AI in diverse domains.
Best practices in using Generative AI
When utilizing Generative AI, it is essential to adhere to best practices to maximize its potential while mitigating associated risks. Here are some recommended best practices:
1. Quality Data Curation
Ensure that the training data used for Generative AI models is diverse, inclusive, and representative of the intended application. Mitigate biases and inaccuracies in the data to enhance the quality of the generated content.
2. Ethical Frameworks
Develop and adhere to ethical frameworks that govern the application of Generative AI, encompassing responsible content generation, transparency, accountability, and measures to combat misuse, misinformation, and deceptive content.
3. User Safeguards
Implement measures to safeguard users against harmful or misleading content generated by AI. Incorporate validation mechanisms, moderation processes, and user awareness initiatives to mitigate potential negative impacts.
4. Bias and Fairness Evaluation
Conduct rigorous assessments to identify and rectify biases in Generative AI outputs. Prioritize fairness, diversity, and inclusivity in the generated content to minimize the propagation of societal biases.
5. Interdisciplinary Collaboration
Foster collaboration between AI specialists, domain experts, ethicists, and legal professionals to comprehensively address the diverse implications of Generative AI, encompassing technical, ethical, and regulatory aspects.
6. Transparent Communication
Clearly communicate the nature of AI-generated content to users, distinguishing it from human-generated content. Disclose the involvement of AI in content creation to promote transparency and informed engagement.
7. Continuous Monitoring and Validation
Establish ongoing monitoring and validation processes to assess the quality, relevance, and ethical implications of generated content. Incorporate user feedback and human validation to iteratively improve content outputs.
8. Regulatory Compliance
Stay apprised of relevant legal and regulatory requirements pertaining to AI-generated content, intellectual property rights, data protection, and consumer protection. Adhere to applicable standards and guidelines.
9. Education and Awareness
Promote understanding and awareness of Generative AI among stakeholders, including users, content creators, and decision-makers. Foster informed discussions on the capabilities, limitations, and ethical considerations of AI-generated content.
By embracing these best practices, organizations and individuals can harness the potential of Generative AI while upholding ethical standards, promoting responsible usage, and fostering trust in AI-generated content.
What to look for in generative AI in 2024 and beyond
In 2024 and beyond, the development of Generative AI is anticipated to undergo significant advancements and introduce new capabilities, shaping its impact on various industries. Here are some key aspects to watch for:
1. Enhanced Creativity and Realism
Generative AI is expected to exhibit increased creativity and realism, allowing for the generation of content that is virtually indistinguishable from human-created work. This could include more natural language generation, lifelike visual synthesis, and innovative artistic expressions.
2. Ethical and Responsible AI Usage
With the growing influence of Generative AI, there will be an increased focus on ethical considerations and responsible usage. Expect to see advancements in AI ethics frameworks, guidelines for content creation, and measures to mitigate the potential misuse of Generative AI.
3. Cross-modal Capabilities
Generative AI models are likely to evolve to integrate cross-modal capabilities, enabling the synergistic generation of content across different modalities such as text, images, and audio. This could give rise to more versatile and holistic content creation systems.
4. Personalization and Customization
Generative AI is anticipated to play a pivotal role in personalizing content and experiences for individuals. This could involve tailored product recommendations, customized media content, and personalized creative outputs based on user preferences and behavior.
5. Improved Human-AI Collaboration
As Generative AI becomes more sophisticated, the focus will shift towards enhancing human-AI collaboration. This entails developing intuitive interfaces and tools that enable seamless interaction between users and Generative AI systems, fostering a symbiotic creative relationship.
6. Domain-specific Applications
Industries such as healthcare, architecture, and manufacturing are expected to adopt Generative AI for domain-specific applications. This could involve the generation of custom medical treatments, automated design prototyping, and optimization of manufacturing processes through AI-generated solutions.
7. Continued Research and Innovation
Research in Generative AI is forecasted to continue at a rapid pace, leading to breakthroughs in understanding AI creativity, novel training methodologies, and the development of more efficient generative models.
Conclusion
Generative AI is a powerful technology that provides businesses with a lot of opportunities to improve their marketing and data analysis processes. It allows companies to automate the creation of high-quality and engaging content while optimizing their cost, saving time, labor, and resources.
Despite some of its challenges, the potential applications of Generative AI are vast, and we can only expect the technology to improve tremendously over the next few years.
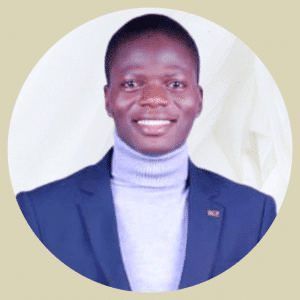
I’m a Digital Marketing Expert, and I love to help businesses gain visibility and scale in online businesses using digital marketing strategies.
Share via: